[TOC]
Author: whuanle
Personal Website: https://www.whuanle.cn
Blog: https://www.cnblogs.com/whuanle/
Tutorial Link: https://kafka.whuanle.cn/
This tutorial is about Kafka knowledge, practicing writing Kafka programs in C# while learning about Kafka.
The content of this tutorial was written during the New Year period. Halfway through, the holiday ended and I had to return to work, so I organized the completed parts and will not continue writing.
1. Setting Up Kafka Environment
The content of this chapter is relatively simple. We will quickly deploy a single-node Kafka or a Kafka cluster using Docker. In the subsequent chapters, we will use the already deployed Kafka instance for experiments and, through continuous experiments, gradually understand the knowledge points of Kafka and master the use of the client.
Here, I provide both single-node and cluster deployment methods, but for the sake of learning in the subsequent chapters, please deploy Kafka in cluster mode.
Installing docker-compose
Using docker-compose to deploy Kafka can reduce a lot of unnecessary trouble; with just one script, deployment can be completed, saving time on setup.
Installing docker-compose is quite simple; you can directly download the binary executable file.
INSTALLPATH=/usr/local/bin
sudo curl -L "https://github.com/docker/compose/releases/download/1.29.2/docker-compose-$(uname -s)-$(uname -m)" -o ${INSTALLPATH}/docker-compose
sudo chmod +x ${INSTALLPATH}/docker-compose
docker-compose --version
If the system does not map the
/usr/local/bin/
path, after executing the command, if you find that thedocker-compose
command cannot be found, please download the file to/usr/bin
, replacingINSTALLPATH=/usr/local/bin
withINSTALLPATH=/usr/bin
.
Deploying Single-node Kafka
Create a docker-compose.yml
file with the following content:
---
version: '3'
services:
zookeeper:
image: confluentinc/cp-zookeeper:7.3.0
container_name: zookeeper
environment:
ZOOKEEPER_CLIENT_PORT: 2181
ZOOKEEPER_TICK_TIME: 2000
broker:
image: confluentinc/cp-kafka:7.3.0
container_name: broker
ports:
- "9092:9092"
depends_on:
- zookeeper
environment:
KAFKA_BROKER_ID: 1
KAFKA_ZOOKEEPER_CONNECT: 'zookeeper:2181'
KAFKA_LISTENER_SECURITY_PROTOCOL_MAP: PLAINTEXT:PLAINTEXT,PLAINTEXT_INTERNAL:PLAINTEXT
KAFKA_ADVERTISED_LISTENERS: PLAINTEXT://192.168.3.156:9092,PLAINTEXT_INTERNAL://broker:29092
KAFKA_OFFSETS_TOPIC_REPLICATION_FACTOR: 1
KAFKA_TRANSACTION_STATE_LOG_MIN_ISR: 1
KAFKA_TRANSACTION_STATE_LOG_REPLICATION_FACTOR: 1
volumes:
- /data/kafka/broker/logs:/opt/kafka/logs
- /var/run/docker.sock:/var/run/docker.sock
Please replace the IP in
PLAINTEXT://192.168.3.156
.
Then execute the command to start deploying the application:
docker-compose up -d
Next, install Kafdrop, which is a Kafka management interface that allows easy access to certain information.
docker run -d --rm -p 9000:9000 \
-e JVM_OPTS="-Xms32M -Xmx64M" \
-e KAFKA_BROKERCONNECT=192.168.3.156:9092 \
-e SERVER_SERVLET_CONTEXTPATH="/" \
obsidiandynamics/kafdrop
Deploying Kafka Cluster
There are many deployment methods for Kafka clusters, and they differ in their configuration parameters (environment variables). Here, I provide only the quick deployment parameters I use; readers can refer to the official documentation for custom configurations.
Some important environment variable explanations in my deployment script are as follows:
KAFKA_BROKER_ID
: The id of the current Broker instance; Broker ids must be unique;KAFKA_NUM_PARTITIONS
: The number of partitions for the default Topic; defaults to 1. If this configuration is set, automatically created Topics will have their partition counts set according to this value.KAFKA_DEFAULT_REPLICATION_FACTOR
: The default number of replicas for Topic partitions;KAFKA_ZOOKEEPER_CONNECT
: The Zookeeper address;KAFKA_LISTENERS
: The IP on which the Kafka Broker instance listens;KAFKA_ADVERTISED_LISTENERS
: How to access the current instance from the outside, used for Zookeeper monitoring;
Create a docker-compose.yml
file with the following content:
---
version: '3'
services:
zookeeper:
image: confluentinc/cp-zookeeper:7.3.0
container_name: zookeeper
environment:
ZOOKEEPER_CLIENT_PORT: 2181
ZOOKEEPER_TICK_TIME: 2000
kafka1:
image: confluentinc/cp-kafka:7.3.0
container_name: broker1
ports:
- 19092:9092
depends_on:
- zookeeper
environment:
KAFKA_BROKER_ID: 1
KAFKA_NUM_PARTITIONS: 3
KAFKA_DEFAULT_REPLICATION_FACTOR: 2
KAFKA_ZOOKEEPER_CONNECT: 'zookeeper:2181'
KAFKA_LISTENERS: PLAINTEXT://0.0.0.0:9092
KAFKA_ADVERTISED_LISTENERS: PLAINTEXT://192.168.3.158:19092
volumes:
- /data/kafka/broker1/logs:/opt/kafka/logs
- /var/run/docker.sock:/var/run/docker.sock
kafka2:
image: confluentinc/cp-kafka:7.3.0
container_name: broker2
ports:
- 29092:9092
depends_on:
- zookeeper
environment:
KAFKA_BROKER_ID: 2
KAFKA_NUM_PARTITIONS: 3
KAFKA_DEFAULT_REPLICATION_FACTOR: 2
KAFKA_ZOOKEEPER_CONNECT: 'zookeeper:2181'
KAFKA_LISTENERS: PLAINTEXT://0.0.0.0:9092
KAFKA_ADVERTISED_LISTENERS: PLAINTEXT://192.168.3.158:29092
volumes:
- /data/kafka/broker2/logs:/opt/kafka/logs
- /var/run/docker.sock:/var/run/docker.sock
kafka3:
image: confluentinc/cp-kafka:7.3.0
container_name: broker3
ports:
- 39092:9092
depends_on:
- zookeeper
environment:
KAFKA_BROKER_ID: 3
KAFKA_NUM_PARTITIONS: 3
KAFKA_DEFAULT_REPLICATION_FACTOR: 2
KAFKA_ZOOKEEPER_CONNECT: 'zookeeper:2181'
KAFKA_LISTENERS: PLAINTEXT://0.0.0.0:9092
KAFKA_ADVERTISED_LISTENERS: PLAINTEXT://192.168.3.158:39092
volumes:
- /data/kafka/broker3/logs:/opt/kafka/logs
- /var/run/docker.sock:/var/run/docker.sock
Since all three Broker instances are on the same virtual machine, different ports are exposed to avoid conflicts.
Then execute the command to start deploying the application:
docker-compose up -d
Next, deploy Kafdrop:
docker run -d --rm -p 9000:9000 \
-e JVM_OPTS="-Xms32M -Xmx64M" \
-e KAFKA_BROKERCONNECT=192.168.3.158:19092,192.168.3.158:29092,192.168.3.158:39092 \
-e SERVER_SERVLET_CONTEXTPATH="/" \
obsidiandynamics/kafdrop
Now, the Kafka environment and management panel have been set up.
2. Kafka Concepts
In this chapter, I will introduce some basic concepts of Kafka. The content in this document is my personal understanding and summary, and there may be errors or other issues; any questions are welcome.
Basic Concepts
A simple structure diagram showing Producing Message
-> Saved to Broker
-> Consuming Message
is as follows:
Here, four objects appear:
Producer: The client that produces Messages;
Consumer: The client that consumes Messages;
Topic: A logical entity;
Message: A data entity;
Of course, each object in the diagram is complex by itself. For the sake of learning, I have drawn a simple diagram; now let's start from the simplest structure to understand these things.
The diagram is quite simple. In Kafka, there are multiple Topics, and the Producer can produce a message to a specified Topic, while the Consumer can consume messages from a specified Topic.
Both Producer and Consumer are client applications; however, they differ in function. In theory, Kafka's client libraries are often written in the same module for both functionalities. For example, in C# with confluent-kafka-dotnet
, it has APIs for both producers and consumers.
Then, there is the Message, which mainly consists of:
Key
Value
Other Metadata
Where Value is where we customize the message content.
Regarding Messages, we can keep our understanding simple for now; we will continue to delve into this topic in subsequent chapters.
In Kafka, each Kafka instance is called a Broker, and each Broker can store multiple Topics. Each Topic can be divided into multiple partitions, and the data stored in each partition is different. These partitions can reside in the same Broker or be spread across different Brokers.
A Broker can store different partitions of different Topics, as well as multiple partitions of the same Topic.
If a Topic has multiple partitions, generally speaking, its concurrency will increase. By increasing the number of partitions, cluster load balancing can be achieved. In most cases, for effective load balancing, the partitions need to be distributed across different Brokers; otherwise, if all partitions reside in the same Broker, the bottleneck will be on that single machine.
If the number of Broker instances is smaller but the Topic has multiple partitions, those partitions will be deployed to the same Broker.
Topic partitions can effectively increase the concurrency of producers or consumers because messages stored in different partitions can be pushed to multiple partitions simultaneously, which is faster than pushing messages to just one partition.
As mentioned earlier, each Message has Key and Value, and Topics can store a Message in different partitions based on the Message's Key. Of course, we can also specify a partition when producing a message.
Partitions can enhance concurrency; however, if a Broker fails, the data will be lost. What can we do about that?
In Kafka, partitions can have multiple replicas, and these replicas are not located on the same Broker. When a Broker fails, these partitions can utilize replicas on other Brokers to recover.
Reminder
Page 21 of the "Kafka: The Definitive Guide (2nd Edition)" provides guidance on how to reasonably set partition numbers, along with the advantages and disadvantages of partitions.
About Kafka Command-Line Tools
Having introduced some simple concepts of Kafka, we can use Kafka scripts to conduct experiments for better understanding.
Open one of the Kafka containers (using the docker exec
command to enter the container), and then execute the command to view the built-in binary scripts:
ls -lah /usr/bin/ | grep kafka
You can see that there are many CLI tools available inside. The documentation for each CLI tool can be found here:
https://docs.cloudera.com/runtime/7.2.10/kafka-managing/topics/kafka-manage-basics.html
Below, I will introduce the usage of some CLI tools.
Topic Management
kafka-topics
is the CLI tool used for managing Topics. kafka-topics
provides basic operations as follows:
- Operations:
--create
: Create a Topic;--alter
: Alter the Topic, modify partition count, etc.;--config
: Modify Topic-related configurations;--delete
: Delete the Topic;
When managing Topics, we can set Topic configurations. The format for storing configurations is default.replication.factor
, and if using the CLI tool, an example of the parameter passed would be --replication-factor
; thus, the parameter names can differ when using different tools to operate on Topics.
All configuration parameters for Topics can be found in the official documentation:
https://kafka.apache.org/090/documentation.html
Common parameters for kafka-topics
include:
--partitions
: The number of partitions, indicating how many partitions this Topic is divided into;--replication-factor
: The number of replicas, indicating how many replicas each partition has; the number of replicas must be less than or equal to the number of Brokers;--replica-assignment
: Specify the replica assignment plan; cannot be used simultaneously with--partitions
or--replication-factor
;--list
: List the active Topics;--describe
: Query the information of the Topic.
Here is a command to manually create a Topic using the CLI, setting the partitions and partition replicas.
kafka-topics --create --bootstrap-server 192.168.3.158:19092 \
--replication-factor 3 \
--partitions 3 \
--topic hello-topic
When using the CLI, you can connect to a Kafka instance through the
--bootstrap-server
configuration or connect to Zookeeper via--zookeeper
, allowing the CLI to automatically find the Kafka instance to execute the command.
View the detailed information of the Topic:
kafka-topics --describe --bootstrap-server 192.168.3.158:19092 --topic hello-topic
Topic: hello-topic TopicId: r3IlKv8BSMaaoaT4MYG8WA PartitionCount: 3 ReplicationFactor: 3 Configs:
Topic: hello-topic Partition: 0 Leader: 3 Replicas: 3,1,2 Isr: 3,1,2
Topic: hello-topic Partition: 1 Leader: 1 Replicas: 1,2,3 Isr: 1,2,3
Topic: hello-topic Partition: 2 Leader: 2 Replicas: 2,3,1 Isr: 2,3,1
You can see that the created partitions are evenly distributed across different Broker instances. We will discuss Replicas and related topics in later chapters.
You can also open Kafdrop to check the Topic information.
What if a Topic has more partitions than there are Brokers? As mentioned earlier, if the number of partitions is large, some Brokers may have multiple partitions of the same Topic.
下面我们来实验验证一下:
kafka-topics --create --bootstrap-server 192.168.3.158:19092 \
--replication-factor 2 \
--partitions 4 \
--topic hello-topic1
可以看到,Broker 2,分到了 hello-topic1
的两个分区。
使用 C# 创建分区
客户端库中可以利用接口管理主题,如 C# 的 confluent-kafka-dotnet,使用 C# 代码创建 Topic 的示例如下:
static async Task Main()
{
var config = new AdminClientConfig
{
BootstrapServers = "192.168.3.158:19092"
};
using (var adminClient = new AdminClientBuilder(config).Build())
{
try
{
await adminClient.CreateTopicsAsync(new TopicSpecification[] {
new TopicSpecification { Name = "hello-topic2", ReplicationFactor = 3, NumPartitions = 2 } });
}
catch (CreateTopicsException e)
{
Console.WriteLine($"An error occured creating topic {e.Results[0].Topic}: {e.Results[0].Error.Reason}");
}
}
}
在 AdminClient 中还有很多方法可以探索。
分区与复制
在前面,我们创建了一个名为 hello-topic
的主题,并且为其设置三个分区,三个副本。
接着,使用 kafka-topics --describe
命令查看一个 Topic 的信息,可以看到:
Topic: hello-topic TopicId: r3IlKv8BSMaaoaT4MYG8WA PartitionCount: 3 ReplicationFactor: 3 Configs:
Topic: hello-topic Partition: 0 Leader: 3 Replicas: 3,1,2 Isr: 3,1,2
Topic: hello-topic Partition: 1 Leader: 1 Replicas: 1,2,3 Isr: 1,2,3
Topic: hello-topic Partition: 2 Leader: 2 Replicas: 2,3,1 Isr: 2,3,1
Partition: 0 Leader: 3 Replicas: 3,1,2 Isr: 3,1,2
这些数字都是指 Broker ID,Broker ID 可以是数字也可以是有英文。
主题的每个分区都有至少一个副本,也就是 --replication-factor
参数必须设置大于大于 1。副本分为 leader 和 follower 两种,每个副本都需要消耗一个存储空间,leader 对外提供读写消息,而 follower 提供冗余备份,leader 会及时将消息增量同步到所有 follower 中。
Partition: 0 Leader: 3 Replicas: 3,1,2
表示分区 0 的副本分布在 ID 为 3
、1
、2
的 Kafka broker 中。
在 hello-topic
主题中,当分区只有一个副本时,或只关注 leader 副本时,leader 副本对应的 Broker 节点位置如下:
Kafka 分配分区到不同的节点有一定的规律,感兴趣的读者可参考 《Kafka 权威指南》第二版或官方文档。
如果设置了多个副本( --replication-factor=3
) 时,leader 副本和 follower 副本的位置如下所示:
分区的副本数量不能大于 Broker 数量,每个 Broker 只能有此分区的一个副本,副本数量范围必须在
[1,{Broker数量}]
中。也就是说,如果集群只有三个 Broker,那么创建的分区,其副本数量必须在[1,3]
范围内。
在不同的副本中,只有 leader 副本能够进行读写,follower 接收从 leader 推送过来的数据,做好冗余备份。
一个分区的所有副本统称为 AR(Assigned Replicas),当 leader 接收到消息时,需要推送到 follower 中,理想情况下,分区的所有副本的数据都是一致的。
但是 leader 同步到 follower 的过程中可能会因为网络拥堵、故障等,导致 follower 在一定时间内未能与 leader 中的数据一致(同步滞后),那么这些副本称为 OSR( Out-Sync Replicas)。
如果副本中的数据为最新的数据,在给定的时间内同步没有出现滞后,那么这些副本称为 ISR。
AR = ISR + OSR
如果 leader 故障,那么剩下的 follower 会重新选举一个 leader;但是如果 leader 接收到生产者的消息后还没有同步到 follower 就故障了,那么这些消息就会丢失。为了避免这种情况,需要生产者设置合理的 ACK,在第四章中会讨论这个问题。
生产者消费者
kafka-console-producer 可以给指定的主题发送消息:
kafka-console-producer --bootstrap-server 192.168.3.158:19092 --topic hello-topic
kafka-console-consumer 则可以从指定主题接收消息:
kafka-console-consumer --bootstrap-server 192.168.3.158:19092 --topic hello-topic \
--group hello-group \
--from-beginning
订阅主题时,消费者需要指定消费者组。可以通过 --group
指定;如果不指定,脚本会自动为我们创建一个消费者组。
kafka-consumer-groups 则可以为我们管理消费者组,例如查看所有的消费者组:
kafka-consumer-groups --bootstrap-server 192.168.3.158:19092 --list
查看消费者组详细信息:
kafka-consumer-groups --bootstrap-server 192.168.3.158:19092 --describe --group hello-group
当然,也可以从 Kafdrop 界面中查看消费者组的信息。
这些参数我们现在可以先跳过。
C# 部分并没有重要的内容要说,代码可以参考:
static async Task Main()
{
var config = new AdminClientConfig
{
BootstrapServers = "192.168.3.158:19092"
};
using (var adminClient = new AdminClientBuilder(config).Build())
{
var groups = adminClient.ListGroups(TimeSpan.FromSeconds(10));
foreach (var item in groups)
{
Console.WriteLine(item.Group);
}
}
}
对于消费者组来说,我们需要关注以下参数:
-
state
:消费者组的状态; -
members
:消费者组成员; -
offsets
: ACK 偏移量;
修改配置
可以使用 kafka-configs 工具设置、描述或删除主题属性。
查看主题属性描述:
kafka-configs --bootstrap-server [HOST:PORT] --entity-type topics --entity-name [TOPIC] --describe
kafka-configs --bootstrap-server 192.168.3.158:19092 --entity-type topics --entity-name hello-topic --describe
使用 --alter
参数后,可以添加、修改或删除主题属性,命令格式:
kafka-configs --bootstrap-server [HOST:PORT] --entity-type topics --entity-name [TOPIC] --alter --add-config [PROPERTY NAME]=[VALUE]
kafka-configs --bootstrap-server [HOST:PORT] --entity-type topics --entity-name [TOPIC] --alter --delete-config [PROPERTY_NAME]
例如 Kafka 默认限制发送的消息最大为 1MB,为了修改这个限制,可以使用以下命令:
kafka-configs --bootstrap-server 192.168.3.158:19092 --entity-type topics --entity-name hello-topic --alter --add-config 'max.message.bytes=1048576'
其中还有很多参数,请参考:
https://kafka.apache.org/10/documentation.html#topicconfigs
此外,我们还可以通过 kafka-configs 查看 Broker 的配置:
kafka-configs --bootstrap-server 192.168.3.158:19092 --describe --broker 1
3, Kafka .NET 基础
在第一章中,笔者介绍了如何部署 Kafka;在第二章中,笔者介绍了 Kafka 的一些基础知识;在本章中,笔者将介绍如何使用 C# 编写程序连接 kafka,完成生产和消费过程。
在第二章的时候,我们已经使用到了 confluent-kafka-dotnet
,通过 confluent-kafka-dotnet
编写代码调用 Kafka 的接口,去管理主题。
confluent-kafka-dotnet
其底层使用了一个 C 语言编写的库 librdkafka,其它语言编写的 Kafka 客户端库也是基于 librdkafka 的,基于 librdkafka 开发客户端库,官方可以统一维护底层库,不同的编程语言可以复用代码,还可以利用 C 语言编写的库提升性能。
此外,因为不同的语言都使用了相同的底层库,也使用了相同的接口,因此其编写的客户端库接口看起来也会十分接近。大多数情况下,Java 和 C# 使用 Kafka 的代码是比较相近的。
接着说一下 confluent-kafka-dotnet
,Github 仓库中对这个库的其中一个特点介绍是:
- High performance : confluent-kafka-dotnet 是一个轻量级的程序包装器,它包含了一个精心调优的 C 语言写的 librdkafka 库。
Library dkafka 是 Apache Kafka 协议的 C 库实现,提供了 Producer、 Consumer 和 Admin 客户端。它的设计考虑到信息传递的可靠性和高性能,目前的性能超过 100万条消息/秒 的生产和 300万条消息/秒 的消费能力(原话是:current figures exceed 1 million msgs/second for the producer and 3 million msgs/second for the consumer)。
现在,这么牛逼的东西,到 nuget 直接搜索 Confluent.Kafka 即可使用。
回归正题,下面笔者将会介绍如果使用 C# 编写生产者、消费者程序。在本章中,我们只需要学会怎么用就行,大概了解过程,而不必深究参数配置,也不必细究代码的功能或作用,在后面的章节中,笔者会详细介绍的。
生产者
编写生产者程序大概可以分为两步,第一步是定义 ProducerConfig 配置,里面是关于生产者的各种配置,例如 Broker 地址、发布消息重试次数、缓冲区大小等;第二步是定义发布消息的过程。例如要发布什么内容、如何记录错误消息、如何拦截异常、自定义消息分区等。
下面是生产者代码的示例:
using Confluent.Kafka;
using System.Net;
public class Program
{
static void Main()
{
var config = new ProducerConfig
{
BootstrapServers = "host1:9092",
...
};
using (var producer = new ProducerBuilder<Null, string>(config).Build())
{
...
}
}
}
如果要将消息推送到 Kafka,那么代码是这样写的:
var result = await producer.ProduceAsync("weblog", new Message<Null, string> { Value="a log message" });
Value
就是消息的内容。其实一条消息的结构比较复杂的,除了 Value ,还有 Key 和各种元数据,这个在后面的章节中我们再讨论。
下面是发布一条消息的实际代码示例:
using Confluent.Kafka;
using System.Net;
public class Program
{
static async Task Main()
{
var config = new ProducerConfig
{
BootstrapServers = "192.168.3.156:9092"
};
using (var producer = new ProducerBuilder<Null, string>(config).Build())
{
var result = await producer.ProduceAsync("weblog", new Message<Null, string> { Value = "a log message" });
}
}
}
运行这段代码后,可以打开 kafdrop 面板查看主题信息。
如果我们断点调试 ProduceAsync
后的内容,可以看到有比较多的信息,例如:
这些信息记录了当前消息是否被 Broker 接收并确认(ACK),该条消息被推送到哪个 Broker 的哪个分区中,消息偏移量数值又是什么。
当然,这里暂时不需要关注这个。
批量生产
这一节中,我们来了解如何通过代码批量推送消息到 Broker。
下面是翻译后的内容:
Here is an example code:
using Confluent.Kafka;
using System.Net;
public class Program
{
static async Task Main()
{
var config = new ProducerConfig
{
BootstrapServers = "192.168.3.156:9092"
};
using (var producer = new ProducerBuilder<Null, string>(config).Build())
{
for (int i = 0; i < 10; ++i)
{
producer.Produce("my-topic", new Message<Null, string> { Value = i.ToString() }, handler);
}
}
// Prevent the program from exiting automatically
Console.ReadKey();
}
public static void handler(DeliveryReport<Null, string> r)
{
Console.WriteLine(!r.Error.IsError
? $"Delivered message to {r.TopicPartitionOffset}"
: $"Delivery Error: {r.Error.Reason}");
}
}
You can see that batch messaging uses Produce
, while the asynchronous code we used earlier employed ProduceAsync
.
Both methods are asynchronous, but the Produce
method maps more directly to the underlying librdkafka API, allowing for high-performance batching of messages in librdkafka. In contrast, ProduceAsync
is a C# implementation of asynchronous calls, which is less overhead compared to Produce
, but ProduceAsync
is still very high-performance—it can generate hundreds of thousands of messages per second on typical hardware.
If we discuss the most intuitive difference, it lies in the return results of the two methods.
From the definition:
Task<DeliveryResult<TKey, TValue>> ProduceAsync(string topic, Message<TKey, TValue> message, ...);
void Produce(string topic, Message<TKey, TValue> message, Action<DeliveryReport<TKey, TValue>> deliveryHandler = null);
ProduceAsync
allows you to directly obtain a Task, then wait for that Task to get the response result.
On the other hand, Produce
does not directly yield a result; instead, it gets the delivery result via a callback executed by librdkafka.
Since Produce
operates asynchronously at the framework layer but does not return a Task, it cannot be await
ed. To prevent the lifespan of the producer
from ending before the batch message processing is complete, you need to use something like producer.Flush(TimeSpan.FromSeconds(10))
to wait for the batch message delivery to finish.
Calling the Flush
method makes all buffered records immediately available for sending, and will block until requests associated with those records are complete.
Flush
has two overloads:
int Flush(TimeSpan timeout);
void Flush(CancellationToken cancellationToken = default(CancellationToken));
The int Flush()
method will wait for the specified time, and if the time elapses with some messages in the queue not sent, it will return the number of messages that were not successfully sent.
Here is the example code:
using Confluent.Kafka;
using System.Net;
public class Program
{
static async Task Main()
{
var config = new ProducerConfig
{
BootstrapServers = "192.168.3.156:9092"
};
using (var producer = new ProducerBuilder<Null, string>(config).Build())
{
for (int i = 0; i < 10; ++i)
{
producer.Produce("my-topic", new Message<Null, string> { Value = i.ToString() }, handler);
}
// Wait for only 10s
var count = producer.Flush(TimeSpan.FromSeconds(10));
// or use
// void Flush(CancellationToken cancellationToken = default(CancellationToken));
}
// Prevent the program from exiting automatically
Console.ReadKey();
}
public static void handler(DeliveryReport<Null, string> r)
{
Console.WriteLine(!r.Error.IsError
? $"Delivered message to {r.TopicPartitionOffset}"
: $"Delivery Error: {r.Error.Reason}");
}
}
If you stop the Kafka service, the client will definitely fail to send messages. What happens when we use the batch sending code?
You can stop all Brokers or set an incorrect address for the BootstrapServers
parameter, then run the program; you will find that producer.Flush(TimeSpan.FromSeconds(10));
will wait for 10s, but the handler will not trigger.
You can see that if you use batch messaging, you need to be cautious about using Flush
; the program will not throw an error even if it cannot connect to the Broker.
Therefore, when we use batch messages, it is crucial to monitor the connection status with the Broker and handle the failure count returned by Flush
.
var result = producer.Flush(TimeSpan.FromSeconds(10));
Console.WriteLine(result);
Using Tasks.WhenAll
As mentioned earlier, using the Produce
method for batch message sending allows for batch commits provided by the framework itself. We can also utilize Tasks.WhenAll
to implement batch commits and retrieve results, although its performance is not as good as that of produce - Flush
.
Here is the example code:
using (var producer = new ProducerBuilder<Null, string>(config).Build())
{
List<Task> tasks = new();
for (int i = 0; i < 10; ++i)
{
var task = producer.ProduceAsync("my-topic", new Message<Null, string> { Value = i.ToString() });
tasks.Add(task);
}
await Task.WhenAll(tasks.ToArray());
}
How to Conduct Performance Testing
How good is the performance of produce - Flush
?
We can use BenchmarkDotNet to test the performance, evaluating the time and memory consumed when pushing different message quantities. Since various factors such as the server's CPU, memory, disk speed, as well as network bandwidth and latency between the client and the server significantly affect message throughput, it is necessary to write code for performance testing to assess the performance required for client and server operations.
Here is the example code:
using Confluent.Kafka;
using System.Net;
using System.Security.Cryptography;
using BenchmarkDotNet.Attributes;
using BenchmarkDotNet.Running;
using BenchmarkDotNet.Jobs;
public class Program
{
static void Main()
{
var summary = BenchmarkRunner.Run<KafkaProduce>();
}
}
[SimpleJob(RuntimeMoniker.Net70)]
[SimpleJob(RuntimeMoniker.NativeAot70)]
[RPlotExporter]
public class KafkaProduce
{
// Batch message size
[Params(1000, 10000,100000)]
public int N;
private ProducerConfig _config;
[GlobalSetup]
public void Setup()
{
_config = new ProducerConfig
{
BootstrapServers = "192.168.3.156:9092"
};
}
[Benchmark]
public async Task UseAsync()
{
using (var producer = new ProducerBuilder<Null, string>(_config).Build())
{
List<Task> tasks = new();
for (int i = 0; i < N; ++i)
{
var task = producer.ProduceAsync("ben1-topic", new Message<Null, string> { Value = i.ToString() });
tasks.Add(task);
}
await Task.WhenAll(tasks);
}
}
[Benchmark]
public void UseLibrd()
{
using (var producer = new ProducerBuilder<Null, string>(_config).Build())
{
for (int i = 0; i < N; ++i)
{
producer.Produce("ben2-topic", new Message<Null, string> { Value = i.ToString() }, null);
}
producer.Flush(TimeSpan.FromSeconds(60));
}
}
}
In the sample code, the author records the time taken as well as the GC statistics.
The results of pinging the server and the BenchmarkDotNet performance test are as follows:
Pinging 192.168.3.156 with 32 bytes of data:
Reply from 192.168.3.156: bytes=32 time=1ms TTL=64
Reply from 192.168.3.156: bytes=32 time=2ms TTL=64
Reply from 192.168.3.156: bytes=32 time=2ms TTL=64
Reply from 192.168.3.156: bytes=32 time=1ms TTL=64
| Method | Job | Runtime | N | Mean | Error | StdDev | Gen0 | Gen1 | Gen2 | Allocated |
| -------- | ------------- | ------------- | ------ | -------: | ------: | -------: | ---------: | --------: | --------: | -----------: |
| UseAsync | .NET 7.0 | .NET 7.0 | 1000 | 125.1 ms | 2.21 ms | 2.17 ms | - | - | - | 1055.43 KB |
| UseLibrd | .NET 7.0 | .NET 7.0 | 1000 | 124.7 ms | 2.26 ms | 2.12 ms | - | - | - | 359.18 KB |
| UseAsync | NativeAOT 7.0 | NativeAOT 7.0 | 1000 | 124.8 ms | 1.83 ms | 1.62 ms | - | - | - | 1055.43 KB |
| UseLibrd | NativeAOT 7.0 | NativeAOT 7.0 | 1000 | 125.1 ms | 1.76 ms | 1.64 ms | - | - | - | 359.18 KB |
| UseAsync | .NET 7.0 | .NET 7.0 | 10000 | 143.9 ms | 3.70 ms | 10.86 ms | 1250.0000 | 750.0000 | 250.0000 | 10577.22 KB |
| UseLibrd | .NET 7.0 | .NET 7.0 | 10000 | 140.6 ms | 2.74 ms | 4.80 ms | 250.0000 | - | - | 3523.29 KB |
| UseAsync | NativeAOT 7.0 | NativeAOT 7.0 | 10000 | 145.7 ms | 3.25 ms | 9.59 ms | 1250.0000 | 750.0000 | 250.0000 | 10577.22 KB |
| UseLibrd | NativeAOT 7.0 | NativeAOT 7.0 | 10000 | 140.6 ms | 2.78 ms | 5.56 ms | 250.0000 | - | - | 3523.29 KB |
| UseAsync | .NET 7.0 | .NET 7.0 | 100000 | 407.3 ms | 7.17 ms | 9.58 ms | 13000.0000 | 7000.0000 | 2000.0000 | 105185.91 KB |
| UseLibrd | .NET 7.0 | .NET 7.0 | 100000 | 259.7 ms | 5.72 ms | 16.78 ms | 4000.0000 | - | - | 35164.82 KB |
| UseAsync | NativeAOT 7.0 | NativeAOT 7.0 | 100000 | 419.8 ms | 8.31 ms | 13.19 ms | 14000.0000 | 8000.0000 | 2000.0000 | 105194.3 KB |
| UseLibrd | NativeAOT 7.0 | NativeAOT 7.0 | 100000 | 255.3 ms | 6.31 ms | 18.62 ms | 4000.0000 | - | - | 35164.72 KB |
It can be seen that using librdkafka for batch sending performs better than using Task.WhenAll
, especially when dealing with a large number of messages.
However, the significance of these performance testing results is limited; it mainly serves to help readers understand how to use BenchmarkDotNet for performance testing, in order to evaluate the throughput that can be achieved in the current environment during message pushing from the client to the Broker.
Consumption
After producing messages, the next step is to write a consumer program to handle the messages. The consumption code is divided into two parts: ConsumerConfig
configuration and the consumption itself. Here is the example code:
using System.Collections.Generic;
using Confluent.Kafka;
...
var config = new ConsumerConfig
{
// The configurations here will be introduced in later chapters, skipping this part for now
BootstrapServers = "host1:9092,host2:9092",
GroupId = "foo",
AutoOffsetReset = AutoOffsetReset.Earliest
};
using (var consumer = new ConsumerBuilder<Ignore, string>(config).Build())
{
...
}
The default consumer configuration automatically commits acknowledgments (ACK), so there is no need to write code to confirm messages after consumption. Thus, the consumer sample code is as follows:
using Confluent.Kafka;
using System.Net;
public class Program
{
static void Main()
{
var config = new ConsumerConfig
{
BootstrapServers = "192.168.3.156:9092",
GroupId = "test1",
AutoOffsetReset = AutoOffsetReset.Earliest
};
CancellationTokenSource source = new CancellationTokenSource();
using (var consumer = new ConsumerBuilder<Ignore, string>(config).Build())
{
// Subscribe to topic
consumer.Subscribe("my-topic");
// Loop to consume
while (!source.IsCancellationRequested)
{
var consumeResult = consumer.Consume(source.Token);
Console.WriteLine(consumeResult.Message.Value);
}
consumer.Close();
}
}
}
In this chapter, we have covered the basics of Kafka .NET. Next, the author will detail how to write producer and consumer code and how to use various parameter configurations.
4. Producer
In the third chapter, we learned about some usage methods of the Kafka C# client and how to write a producer program.
In this chapter, the author will introduce the parameter configurations and API usage methods for the producer program in detail to better apply Kafka in projects and address potential failures.
The following diagram illustrates the process of a producer pushing messages:
Writing a producer using a client library is relatively straightforward; however, the message pushing process is more complex. As shown in the figure above, when a producer pushes a message, the client library first serializes the message into binary using a serializer, then calculates which Broker and which partition the Topic’s message needs to be pushed to using a partitioner.
Next, if pushing a message fails, the client library must also confirm whether to retry, including the number of retries and the time intervals, among others.
Thus, while pushing a message is simple, how to handle faults, ensure messages are not lost, and the producer's configuration require developers to consider the scenario and design reasonable producer program logic.
Regarding the topic "avoiding message loss," in addition to requiring the producer to ensure that messages have been pushed to the Broker, there is also a need to monitor whether the leader replica synchronizes with the follower replicas promptly. Otherwise, even if the client has pushed the message to the Broker, if the Broker's leader has not synchronized the latest message to the follower replicas and it goes down, that message will still be lost. Therefore, the client must also set reasonable ACKs.
This explanation indicates that whether messages will be lost is related not only to the state of the producer but also to the state of the Broker.
Below, the author will detail some configurations and details encountered in daily development when pushing messages with the producer.
Connecting to Broker
The producer connects to the Broker by defining ProducerConfig
, starting with the BootstrapServers
property, where all Broker server addresses are provided in the following format:
host1:9092,host2:9092,...
using Confluent.Kafka;
using System.Net;
public class Program
{
static void Main()
{
var config = new ProducerConfig
{
BootstrapServers = "host1:9092",
...
};
... ...
}
}
If a secure connection is needed, ProducerConfig
can refer to the code below:
var config = new ProducerConfig
{
BootstrapServers = "<your-IP-port-pairs>",
SslCaLocation = "/Path-to/cluster-ca-certificate.pem",
SecurityProtocol = SecurityProtocol.SaslSsl,
SaslMechanism = SaslMechanism.ScramSha256,
SaslUsername = "ickafka",
SaslPassword = "yourpassword",
};
Clients do not need to provide the addresses of all Brokers because, once a connection is established, the producer can retrieve the cluster information and addresses of all Brokers from the connected Brokers. However, it is recommended to fill in at least two Broker addresses, because if the first Broker address is unavailable, the client can still fetch the current cluster information from other Brokers, avoiding complete failure to connect to the server.
For instance, if the server has three Brokers and the client only fills in one BootstrapServers
address, the messages will still be automatically pushed to the corresponding partitions.
static async Task Main()
{
var config = new ProducerConfig
{
BootstrapServers = "192.168.3.158:19092"
};
using (var producer = new ProducerBuilder<string, string>(config).Build())
{
var r1 = await producer.ProduceAsync("hello-topic", new Message<string, string> { Key = "a", Value = "a log message" });
var r2 = await producer.ProduceAsync("hello-topic", new Message<string, string> { Key = "b", Value = "a log message" });
var r3 = await producer.ProduceAsync("hello-topic", new Message<string, string> { Key = "c", Value = "a log message" });
var r4 = await producer.ProduceAsync("hello-topic", new Message<string, string> { Key = "d", Value = "a log message" });
Console.WriteLine($@"
r1 Status:{r1.Status},Partition:{r1.Partition}
r2 Status:{r2.Status},Partition:{r2.Partition}
r3 Status:{r3.Status},Partition:{r3.Partition}
r4 Status:{r4.Status},Partition:{r4.Partition}
");
}
}
It can be seen that even if only one Broker is specified, the messages can still be correctly partitioned.
Key partitioning
This section will introduce the use of Key.
A topic named hello-topic
has been created beforehand, with 3 partitions and 3 replicas, and the command to create it is as follows:
kafka-topics --create --bootstrap-server 192.168.3.158:19092 \
--replication-factor 2 \
--partitions 3 \
--topic hello-topic
In previous chapters, the author introduced how to write producers and push messages. However, the code was relatively simple, and only the Value
was set.
new Message<Null, string> { Value = "a log message" }
Next is the issue regarding partitions.
Firstly, the partitioner determines which partition the current message will be pushed to, and the partitioner resides in the client.
When pushing a message, we can explicitly specify which partition to push the message to; if no partition is explicitly specified, the partitioner will determine the partition based on the Key.
If a message does not have a Key set (i.e., Key
is null
), then those messages without a Key will be evenly distributed across all partitions, following a sequence like p0 => p1 => p2 => p0
.
Next, the author will introduce the use of Key
.
After creating the topic, let's look at the producer builder in C# code and the definition of Message<TKey, TValue>
.
Both ProducerBuilder<TKey, TValue>
and Message<TKey, TValue>
share the same generic parameters.
public class ProducerBuilder<TKey, TValue>
public class Message<TKey, TValue> : MessageMetadata
{
//
// Summary:
// Gets the message key value (possibly null).
public TKey Key { get; set; }
//
// Summary:
// Gets the message value (possibly null).
public TValue Value { get; set; }
}
When writing the code, we need to set the corresponding types for Key and Value.
The sample code for the producer is as follows:
static async Task Main()
{
var config = new ProducerConfig
{
BootstrapServers = "192.168.3.158:19092,192.168.3.158:29092,192.168.3.158:39092"
};
using (var producer = new ProducerBuilder<int, string>(config).Build())
{
var r1 = await producer.ProduceAsync("hello-topic", new Message<int, string> { Key = 1, Value = "a log message" });
var r2 = await producer.ProduceAsync("hello-topic", new Message<int, string> { Key = 2, Value = "a log message" });
var r3 = await producer.ProduceAsync("hello-topic", new Message<int, string> { Key = 3, Value = "a log message" });
var r4 = await producer.ProduceAsync("hello-topic", new Message<int, string> { Key = 4, Value = "a log message" });
Console.WriteLine($@"
r1 Status:{r1.Status},Partition:{r1.Partition}
r2 Status:{r2.Status},Partition:{r2.Partition}
r3 Status:{r3.Status},Partition:{r3.Partition}
r4 Status:{r4.Status},Partition:{r4.Partition}
");
}
}
The response result shows to which partition the message has been pushed.
Next, there is a question: if the same value of Key is pushed to the Broker, will it overwrite the previous message?
Normally, it should not.
There is a
cleanup.policy
parameter for the topic that sets the log retention policy. If the retention policy is compact, only the latest value for each key will be retained.
Next, we can conduct an experiment where we push a total of 20 messages to the Broker with 10 Keys, each repeated twice:
static async Task Main()
{
var config = new ProducerConfig
{
BootstrapServers = "192.168.3.158:19092",
};
using (var producer = new ProducerBuilder<string, string>(config)
.Build())
{
int i = 1;
while (i <= 10)
{
var r1 = await producer.ProduceAsync("same-hello", new Message<string, string> { Key = i.ToString(), Value = "1" });
Console.WriteLine($"id:{r1.Key},status:{r1.Status}");
i++;
}
i = 1;
while (i <= 10)
{
var r1 = await producer.ProduceAsync("same-hello", new Message<string, string> { Key = i.ToString(), Value = "2" });
Console.WriteLine($"id:{r1.Key},status:{r1.Status}");
i++;
}
}
}
Or:
int i = 1; while (i <= 10) { var r1 = await producer.ProduceAsync("same-hello", new Message<string, string> { Key = i.ToString(), Value = "1" }); Console.WriteLine($"id:{r1.Key},status:{r1.Status}"); var r2 = await producer.ProduceAsync("same-hello", new Message<string, string> { Key = i.ToString(), Value = "2" }); Console.WriteLine($"id:{r1.Key},status:{r2.Status}"); i++; }
Then, we can open Kafdrop to see how many messages are in each partition.
As seen, the total number of messages is 20, and although some keys are repeated, the messages are still there and have not been lost.
Next, after opening one of the partitions, we can find that the partitioner still functions normally, with the same keys being routed to the same partition.
Therefore, we need not worry about keys being empty or messages being overwritten with the same keys.
Assessing Message Sending Time
The following outlines the steps for pushing a message.
<br />
。
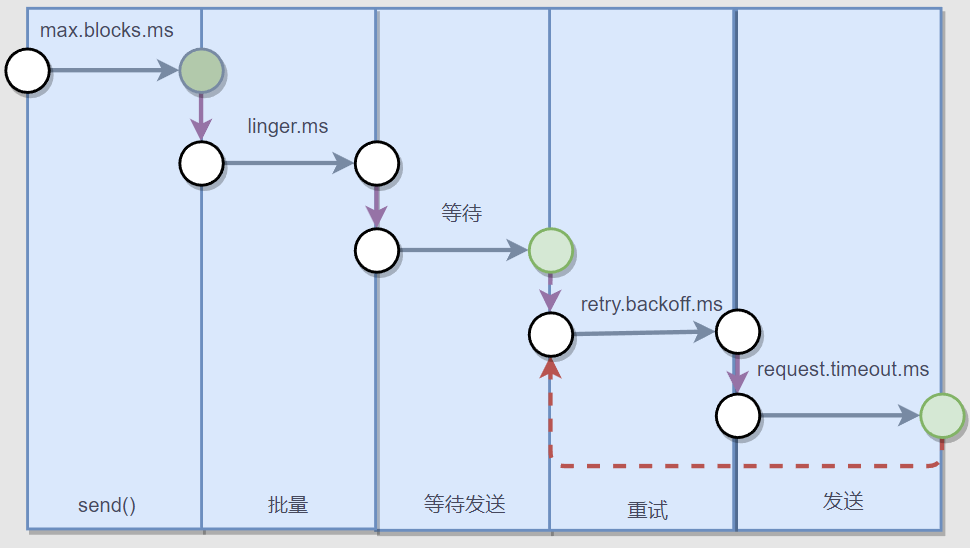
> Here, "batch" refers to the buffer.
The client library involves several time configurations. In "Kafka: The Definitive Guide (2nd Edition)," a time formula is provided:
```csharp
delivery.timeout.ms >= linger.ms + retry.backoff.ms + request.timeout.ms
The delivery.timeout.ms
setting indicates that the total time for putting messages into the buffer, pushing messages to the Broker, receiving an Ack, and retrying must not exceed this range; otherwise, it is considered a timeout.
In C#, there aren't such detailed time configurations, and verifying these time configurations can be quite complex. Therefore, the author provides only a brief overview here; for detailed information on each time configuration, readers can refer to page 41 of "Kafka: The Definitive Guide (2nd Edition)."
Producer Configuration
This section mainly references the article:
https://towardsdatascience.com/10-configs-to-make-your-kafka-producer-more-resilient-ec6903c63e3f
Some images are sourced from this article.
Other reference materials include "Kafka: The Definitive Guide (2nd Edition)."
This section introduces the following producer configurations:
acks
bootstrap.servers
retries
enable.idempotence
max.in.flight.requests.per.connection
buffer.memory
max.block.ms
linger.ms
batch.size
compression.type
Examining the source code of ProducerConfig reveals that each property corresponds to a Kafka configuration item.
Complete producer configuration documentation: https://docs.confluent.io/platform/current/installation/configuration/producer-configs.html#
Next, the author provides a detailed explanation of the configurations that are commonly used in daily development.
acks
The corresponding enumeration in C# is as follows:
public enum Acks
{
None = 0,
Leader = 1,
All = -1
}
Usage example:
var config = new ProducerConfig
{
BootstrapServers = "192.168.3.158:9092",
Acks = Acks.Leader
};
The default value is
Acks.Leader
.
acks
specifies that the producer considers a message successfully written only when a specified number of partition replicas have received the message. By default, as long as the leader replica receives the message, it responds to the client that the message has been successfully written. This helps control the persistence of the sent messages.
Here’s an explanation of the acks
configurations:
acks=0
: This means that the record is immediately added to the socket buffer and is considered sent. If there is a network failure or other reason that prevents the message from being pushed to the Broker, the message will be discarded.acks=1
: As long as the producer receives an acknowledgment from the leader replica, it considers the submission successful. However, if the leader replica crashes, there is still a risk of message loss.acks=all
: The message must wait for acknowledgment from all replicas of the topic after submission, providing the strongest message persistence but increasing latency.
The scenarios involving leaders and followers have been mentioned in Chapters 2 and 3.
The default value for acks
is 1, meaning as long as the producer receives an ack from the leader replica of the topic, it considers the submission successful and proceeds to the next message.
Setting acks=all
ensures that the producer receives acks from all synchronous replicas of the topic before considering the message submitted, providing the strongest message persistence but also requiring more time, leading to higher latency.
The following diagram illustrates the difference between acks=1
and acks=all
.
acks=all
can also be written asacks=-1
.
【Image source: https://towardsdatascience.com/10-configs-to-make-your-kafka-producer-more-resilient-ec6903c63e3f】
bootstrap.servers
This has been mentioned earlier and will not be elaborated upon here.
retries
By default, if message submission fails, the producer does not resend the record, meaning no retries are made; the default retry count is 0.
You can enable message retrying by setting retries = n
, allowing failed messages to be retried n times.
In C#, you can set the maximum retry count through the MessageSendMaxRetries
property of ProducerConfig
.
public int? MessageSendMaxRetries
{
get
{
return GetInt("message.send.max.retries");
}
set
{
SetObject("message.send.max.retries", value);
}
}
【Image source: https://towardsdatascience.com/10-configs-to-make-your-kafka-producer-more-resilient-ec6903c63e3f】
Additionally, you can set the retry backoff interval, which defaults to 100ms.
public int? RetryBackoffMs
{
get
{
return GetInt("retry.backoff.ms");
}
set
{
SetObject("retry.backoff.ms", value);
}
}
enable.idempotence
In simple terms, idempotence is the property of certain operations that allows them to be applied multiple times without changing the result. When enabled, the producer ensures that only one record copy is published to the stream. The default value is false, which means that the producer can write duplicate copies of messages to the stream. To enable idempotence, use the following command:
enable.idempotent=true
When idempotent production is enabled, every message sent by the producer is assigned a sequence number.
In some situations, messages may actually be committed to all synchronous replicas, but due to network issues, the broker fails to send back an ack (for example, one-way communication is allowed only). If we set retry = 3
, the producer will resend messages three times. This may lead to duplicate messages appearing in the topic.
The ideal scenario is to achieve "exactly-once" semantics, where even if the producer resends a message, the consumer should only receive the same message once.
How does it work? Messages are sent in batches, each with a unique sequence number. On the broker side, it tracks the maximum sequence number for each partition. If a batch with a smaller or equal sequence number arrives, the broker will not write that batch into the topic. This also ensures the order of the batches.
【Image source: https://towardsdatascience.com/10-configs-to-make-your-kafka-producer-more-resilient-ec6903c63e3f】
max.in.flight.requests.per.connection
The Connection Kafka Producer Config indicates the maximum number of unacknowledged requests sent on a single connection before blocking the client. The default value is 5.
If retries are enabled and max.in.flight.requests.per.connection
is set to greater than 1, there is a risk of message reordering.
Another important configuration to ensure order is max.in.flight.requests.per.connection
, which defaults to 5. This indicates the number of unacknowledged requests that can be buffered on the producer side. If the number of retries is greater than 1, and the first request fails while the second request succeeds, the first request will be retried, causing a message order mismatch.
Please note that if this setting is greater than 1 and a send fails, there is a risk of message reordering due to retries (if retries are enabled).
If enable.idempotent=true
is not set but you still want to maintain message order, you should configure this setting to 1.
However, if enable.idempotent=true
is already enabled, then explicit definition of this configuration is unnecessary. Kafka will select the appropriate value, as described here.
buffer.memory
buffer.memory
represents the total size in bytes of memory the producer can use to buffer messages awaiting sending to the server.
The default value is 32 MB. If the producer sends records faster than they are transferred to the server, the buffer will eventually run out. Before messages in the buffer can be reduced, other messages must wait to be added to the buffer, which will block the producer from sending messages.
Additionally, there is a max.block.ms
parameter that can be configured to specify the maximum time messages can wait to enter the buffer, with a default of 60s. If messages cannot enter the buffer for an extended time, an exception will be thrown.
【Image source: https://towardsdatascience.com/10-configs-to-make-your-kafka-producer-more-resilient-ec6903c63e3f】
Two additional configurations that can be used are linger.ms
and batch.size
. linger.ms
is the delay time before the buffer sends a batch, with a default value of 0, meaning that even if there is only one message in the batch, it will be sent immediately.
Setting linger.ms
to a higher value can reduce the number of requests sent and allow multiple messages to be sent in a batch, improving throughput, but this will result in more messages being held in memory.
There is a configuration equivalent to linger.ms
, which is batch.size
, representing the maximum number of messages in a single batch.
When either of these two criteria is met, the batch messages will be sent.
batch.size
Whenever multiple records are sent to the same partition, the producer attempts to batch the records together. This way, the performance of both the client and the server can be improved. batch.size
represents the maximum size (in bytes) of a single batch.
Sending multiple records to the same partition can enhance performance through batching. The size represents the maximum size (in bytes) of a single batch.
A small batch size will make batching irrelevant and reduce throughput, while a very large batch size may lead to memory wastage as memory is typically allocated in anticipation of extra records.
compression.type
By default, messages sent by the producer are uncompressed. This parameter can be set to snappy, gzip, lz4, or zstd, specifying which compression algorithm to use before sending messages to the broker. The snappy compression algorithm, invented by Google, consumes less CPU time while providing reasonable performance and a significant compression ratio. This algorithm can be used if performance and network bandwidth are both considerations. The gzip compression algorithm generally consumes more CPU time but offers a higher compression ratio. This algorithm can be chosen if network bandwidth is limited. Using compression can reduce network transmission and storage overhead, which is often the bottleneck when sending messages to Kafka.
Producer Interceptors
The dkafka library has an interceptor API, but you need to write them in C, making state sharing from C# code complex.
https://github.com/confluentinc/confluent-kafka-dotnet/issues/1454
Serializers
There are Key and Value serializers.
.SetKeySerializer(...)
.SetValueSerializer(...)
Essentially, Apache Kafka provides the ability to easily publish and subscribe to record streams. Therefore, we can flexibly create our custom serializers and deserializers, helping in the transmission of different data types.
The process of converting an object into a byte stream for transmission is referred to as serialization. Nevertheless, Apache Kafka stores and transmits these byte arrays in its queues.
However, the opposite of serialization is deserialization. Here, we convert the byte arrays back into the desired data types. That said, Kafka only provides serializers and deserializers for a limited number of data types, such as:
- String
- Long
- Double
- Integer
- Bytes
In other words, before sending the entire message to the broker, the producer needs to know how to use a serializer to convert the message into a byte array. Similarly, to convert the byte array back into an object, the consumer uses a deserializer.
In C#, the Serializers define several default serializers.
Utf8
Null
Int64
Int32
Single
Double
ByteArray
Since converting byte[] to the corresponding type is not complex, the source code for part of the serializers is shown below:
private class Utf8Serializer : ISerializer<string>
{
public byte[] Serialize(string data, SerializationContext context)
{
if (data == null)
{
return null;
}
return Encoding.UTF8.GetBytes(data);
}
}
private class NullSerializer : ISerializer<Null>
{
public byte[] Serialize(Null data, SerializationContext context)
{
return null;
}
}
private class Int32Serializer : ISerializer<int>
{
public byte[] Serialize(int data, SerializationContext context)
{
return new byte[4]
{
(byte)(data >> 24),
(byte)(data >> 16),
(byte)(data >> 8),
(byte)data
};
}
}
If more types need to be supported, you can implement them by inheriting from ISerializer<T>
.
Due to C# having generics, when using new ProducerBuilder<TKey, TValue>
, it will automatically find the suitable ISerializer<T>
from the default serializers. If the type is not among the default, you will need to implement a serializer yourself.
The producer sets the corresponding serializer, and the client can also set the corresponding deserializer to correctly restore the corresponding structure from the Message.
Similarly, there are several default deserializers defined in Deserializers
. Since the configurations for producers and consumers are interconnected, this will not be repeated when explaining consumers.
using (var consumer = new ConsumerBuilder<Ignore, string>(config)
.SetKeyDeserializer(Deserializers.Ignore)
.Build())
{
}
Headers
Headers contain metadata in messages, mainly for adding some data to the message, such as sources or tracking information.
在 C# 中,一个消息的定义如下:
public class MessageMetadata
{
public Timestamp Timestamp { get; set; }
public Headers Headers { get; set; }
}
public class Message<TKey, TValue> : MessageMetadata
{
public TKey Key { get; set; }
public TValue Value { get; set; }
}
我们可以通过在消息的 Headers 中加入自定义的消息,其示例如下:
var message = new Message<Null, string>
{
Value = "666",
Headers = new Headers()
{
{ "Level", Encoding.ASCII.GetBytes("Info")},
{ "IP", Encoding.ASCII.GetBytes("192.168.3.66")}
}
};
var result = await producer.ProduceAsync("my-topic", message);
生产者处理器
SetStatisticsHandler
SetKeySerializer
SetValueSerializer
SetPartitioner
SetDefaultPartitioner
SetErrorHandler
SetLogHandler
Statistics 统计数据
通过将 statistics.interval.ms
配置属性设置一个固定值,library dkafka 可以配置为以固定的时间间隔发出内部指标,也就是说可以定期获取到 Kafka 集群的所有信息。
首先修改生产者配置中的 StatisticsIntervalMs 属性
var config = new ProducerConfig
{
BootstrapServers = "192.168.3.158:19092",
StatisticsIntervalMs = 1000,
};
然后使用 SetStatisticsHandler 设置处理器,其委托定义为:Action<IProducer<TKey, TValue>, string> statisticsHandler
。
委托中一共有两个参数变量,前者 IProducer<TKey, TValue>
就是当前生产者实例,后者 string
是 Json 文本,记录了当前所有 Broker 的所有详细信息。
由于表示的内容很多,读者可以参考:
https://github.com/confluentinc/librdkafka/blob/master/STATISTICS.md
使用实例如下:
static async Task Main()
{
var config = new ProducerConfig
{
BootstrapServers = "192.168.3.158:19092,192.168.3.158:29092,192.168.3.158:39092",
StatisticsIntervalMs = 1000,
};
using (var producer = new ProducerBuilder<int, string>(config)
.SetStatisticsHandler((producer, json) =>
{
Console.WriteLine(producer.Name);
Console.WriteLine(json);
})
.Build())
{
int i = 100;
while (true)
{
Thread.Sleep(1000);
var r1 = await producer.ProduceAsync("hello-topic", new Message<int, string> { Key = i, Value = "a log message" });
i++;
}
}
}
SetPartitioner、SetDefaultPartitioner
由于指定生产者在向 Broker 推送消息时,消息向指定分区写入。
SetPartitioner
的定义如下:
SetPartitioner:
SetPartitioner(string topic, PartitionerDelegate partitioner)
-- PartitionerDelegate:
Partition PartitionerDelegate(string topic, int partitionCount, ReadOnlySpan<byte> keyData, bool keyIsNull);
SetDefaultPartitioner
的定义如下:
SetDefaultPartitioner(PartitionerDelegate partitioner)
SetPartitioner、SetDefaultPartitioner 的区别在于 SetPartitioner 可以对指定的 topic 有效,SetDefaultPartitioner 则对当前生产者中的所有 topic 有效。
代码示例如下:
static async Task Main()
{
var config = new ProducerConfig
{
BootstrapServers = "192.168.3.158:19092,192.168.3.158:29092,192.168.3.158:39092",
StatisticsIntervalMs = 1000,
};
using (var producer = new ProducerBuilder<int, string>(config)
.SetPartitioner("hello-topic", (topic, partitionCount, keyData, keyIsNull) =>
{
return new Partition(0);
})
.SetDefaultPartitioner((topic, partitionCount, keyData, keyIsNull) =>
{
return new Partition(0);
})
.Build())
{
int i = 100;
while (true)
{
Thread.Sleep(1000);
var r1 = await producer.ProduceAsync("hello-topic", new Message<int, string> { Key = i, Value = "a log message" });
i++;
}
}
}
可以看到,现在所有 topic 都向指定的分区 0 写入:
剩下的两个 SetErrorHandler
、SetLogHandler
,用于记录错误日志、普通日志,读者可根据其它资料自行实验,这里笔者就不再赘述了。
using (var producer = new ProducerBuilder<int, string>(config)
.SetErrorHandler((p, err) =>
{
Console.WriteLine($"Producer Name:{p.Name},error:{err}");
})
.SetLogHandler((p, log) =>
{
Console.WriteLine($"Producer Name:{p.Name},log messagge:{JsonSerializer.Serialize(log)}");
})
.Build())
{
}
异常处理和重试
生产者推送消息有三种发送方式:
-
发送并忘记
-
同步发送
-
异步发送
发送消息时,一般有两种异常情况,一种是可重试异常,例如网络故障、Broker 故障等;另一种是不可重试故障,例如服务端限制了单条消息的最大字节数,但是客户端的消息超过了这个限制,此时会直接抛出异常,而不能重试。
using (var producer = new ProducerBuilder<string, string>(config)
.Build())
{
try
{
var r1 = await producer.ProduceAsync("same-hello", new Message<string, string> { Key = "1", Value = "1" });
Console.WriteLine($"id:{r1.Key},status:{r1.Status}");
}
catch (ProduceException<string, string> ex)
{
Console.WriteLine($"Produce error,key:[{ex.DeliveryResult.Key}],errot message:[{ex.Error}],trace:[{ex.StackTrace}]");
}
catch (Exception ex)
{
Console.WriteLine(ex.Message);
}
}
消息发送后会返回 DeliveryResult<TKey, TValue>
,其 Status
字段表示了消息的状态,有三种状态。
// 消息持久状态的枚举。
public enum PersistenceStatus
{
// 消息从未传输到 Broker,或者失败,并出现错误,指示未将消息写入日;应用程序重试可能导致排序风险,但不会造成复制风险。
NotPersisted,
// 消息被传输到代理,但是没有收到确认;应用程序重试有排序和复制的风险。
PossiblyPersisted,
// 消息被写入日志并由 Broker 确认。在发生代理故障转移的情况下,应使用 `acks='all'` 选项使其完全受信任。
Persisted
}
在消息发送失败时,客户端可以进行重试,可以设置重试次数和重试间隔,还可以设置是否重新排序。
是否重新排序可能会对业务产生极大的影响。
例如发送顺序为
[A,B,C,D]
,当客户端发送 A 失败时,如果不允许重新排序,那么客户端会重试 A,A 成功后继续发送[B,C,D]
,这一过程是阻塞的。如果允许重新排序,那么客户端会在稍候对 A 进行重试,而现在先发送
[B,C,D]
;这样可能会导致 Broker 收到的消息顺序是[B,C,D,A]
。
示例代码如下:
static async Task Main()
{
var config = new ProducerConfig
{
BootstrapServers = "192.168.3.158:19092",
// 接收来自所有同步副本的确认
Acks = Acks.All,
// 最大重试次数
MessageSendMaxRetries = 3,
// 重试时间间隔
RetryBackoffMs = 1000,
// 如果不想在重试时对消息重新排序,则设置为 true
EnableIdempotence = true
};
using (var producer = new ProducerBuilder<string, string>(config)
.SetLogHandler((_, message) =>
{
Console.WriteLine($"Facility: {message.Facility}-{message.Level} Message: {message.Message}");
})
.SetErrorHandler((_, e) =>
{
Console.WriteLine($"Error: {e.Reason}. Is Fatal: {e.IsFatal}");
})
.Build())
{
try
{
var result = await producer.ProduceAsync("same-hello", new Message<string, string> { Key = "1", Value = "1" });
Console.WriteLine($"[{result.Key}] 发送状态; {result.Status}");
// 消息没有收到 Broker 的 ACK
if (result.Status != PersistenceStatus.Persisted)
{
// 自动重试失败后,此消息需要手动处理。
}
}
catch (ProduceException<string, string> ex)
{
Console.WriteLine($"Produce error,key:[{ex.DeliveryResult.Key}],errot message:[{ex.Error}],trace:[{ex.StackTrace}]");
}
catch (Exception ex)
{
Console.WriteLine(ex.Message);
}
}
}
Broker 限制速率
在 Kafka 中,生产者、消费者都是客户端,两者都有一个 client.id
,消费者还有一个消费者组的概念,但生产者只有 client.id
,没有其它标识了。
一般来说,并不需要设定 生产者的 client.id
,框架会自动设置,如:
rdkafka#producer-1
var config = new ProducerConfig
{
BootstrapServers = "192.168.3.158:19092",
StatisticsIntervalMs = 1000,
ClientId = "abcdef"
};
新的 client.id
:
abcdef#producer-1
回归正题,在 Kafka 中,可以根据 client.id
,对生产者或消费者进行限制流量,多个客户端(消费者或生产者)可以用同一个 client.id
。或者通过其它认证机制标识客户端身份。
可以通过以下方式表示客户端。
-
user
-
client id
-
user + client id
笔者选择使用最简单的 client.id
做实验。
kafka-configs --alter --bootstrap-server 192.168.3.158:19092 --add-config 'producer_byte_rate=1024,consumer_byte_rate=1024' --entity-type clients --entity-name 'abcdef'
限制 1kb/s。
然后编写使用下面的代码测试,会发现推送消息速度变得很慢。
static async Task Main()
{
var config = new ProducerConfig
{
BootstrapServers = "192.168.3.158:19092,192.168.3.158:29092,192.168.3.158:39092",
StatisticsIntervalMs = 1000,
ClientId = "abcdef"
};
using (var producer = new ProducerBuilder<int, string>(config)
.Build())
{
int i = 1000;
var str = string.Join(",", Enumerable.Range(0, 1024).Select(x => x.ToString("X16")));
while (true)
{
var r1 = await producer.ProduceAsync("hello-topic", new Message<int, string> { Key = i, Value = str });
i++;
Console.WriteLine($"id:{r1.Key},status:{r1.Status}");
}
}
}
5. Consumer
In the producer section of Chapter 4, many features of the producer were introduced, and since many features of the consumer are similar to that of the producer, this chapter will briefly introduce the writing method of the consumer program and some solutions to problems without further elaborating on the consumer parameters.
Consumer and Consumer Group
When creating a consumer, you can specify the group to which the consumer belongs (GroupId
). If not specified, Kafka will assign one by default.
To specify a consumer group C
for the consumer, use the following method:
var config = new ConsumerConfig
{
BootstrapServers = "host1:9092,host2:9092",
GroupId = "C",
AutoOffsetReset = AutoOffsetReset.Earliest
};
The consumer group is a very important configuration.
If a topic has only one partition and there is only one consumer group with only one consumer, the consumption process is as shown below.
All messages in
hello-topic
will be consumed byC-C1
.
A partition can only be consumed by one consumer in a consumer group! In consumer group C, regardless of how many consumers there are, partition 0 can only be consumed by one consumer.
If the C1 consumer program crashes, and the C2 consumer begins to consume, then by default, consumption will start from where the C1 consumer last consumed.
If a topic has multiple consumer groups, then each consumer group can consume all messages from this partition.
Each consumer group has its own consumption record.
If there are multiple consumers in a consumer group, then a partition will only be assigned to one of the consumers.
At this time, C2 has nothing to do.
If the topic has multiple partitions, the partitions will be allocated to the consumers in the consumer group according to certain rules, as shown below, where consumer C1 is assigned to partition 0 and partition 2, while consumer C2 is assigned to partition 1.
In consumer group G, there is only one consumer, so G1 is assigned to all partitions.
Generally, it is best for the number of consumers in a consumer group to match the number of partitions, so that each consumer can consume one partition. Too many consumers will lead to some consumers being unable to consume messages, while too few consumers will cause a single consumer to handle messages from multiple partitions.
After the consumer connects to the Broker, the Broker will assign topic partitions to the consumer.
By default, a consumer's group membership identity is temporary. When a consumer leaves the group, the ownership of the partitions assigned to it will be revoked; when that consumer rejoins, a new member ID and new partitions will be assigned to it through the rebalancing protocol. A unique group.instance.id
can be assigned to the consumer to make it a fixed member of the group.
var config = new ConsumerConfig
{
BootstrapServers = "192.168.3.158:19092",
GroupId = "C",
GroupInstanceId = "C1",
AutoOffsetReset = AutoOffsetReset.Earliest,
};
If two consumers use the same group.instance.id
to join the same group, the second consumer will receive an error informing it that a consumer with the same ID already exists.
Consumption Position
By default, the consumer's AutoOffsetReset
parameter is AutoOffsetReset.Earliest
, which means it will automatically start consuming from the last consumption position of the consumer group.
static void Main()
{
var config = new ConsumerConfig
{
BootstrapServers = "192.168.3.158:19092",
GroupId = "foo",
AutoOffsetReset = AutoOffsetReset.Earliest
};
using (var consumer = new ConsumerBuilder<int, string>(config).Build())
{
consumer.Subscribe("hello-topic");
while (true)
{
var consumeResult = consumer.Consume();
Console.WriteLine(consumeResult.Message.Value);
}
}
}
The definition of AutoOffsetReset is as follows:
public enum AutoOffsetReset
{
Latest,
Earliest,
Error
}
public AutoOffsetReset? AutoOffsetReset
{
get
{
return (AutoOffsetReset?)GetEnum(typeof(AutoOffsetReset), "auto.offset.reset");
}
set
{
SetObject("auto.offset.reset", value);
}
}
Here is a description of the three enumerations:
-
latest
(default) which means consumers will read messages from the tail of the partitionThe latest (default) means consumers will read messages from the tail of the partition, only consuming the latest information, which means the messages received since the consumer went online. This will lead to previous unprocessed messages being ignored.
-
earliest
which means reading from the oldest offset in the partitionThis means reading from the oldest offset in the partition; it will automatically start consuming from the last position the consumer consumed.
-
none
throws an exception to the consumer if no previous offset is found for the consumer's groupIf no previous offset is found for the consumer's group, no exception will be thrown.
You can view the consumption offset in Kafdrop.
Manual Commit
The client can set manual acknowledgment as opposed to automatic message acknowledgment.
var config = new ConsumerConfig
{
BootstrapServers = "192.168.3.158:19092,192.168.3.158:29092,192.168.3.158:39092",
GroupId = "foo",
// Whether to auto commit, does not affect self-positioning consumption
EnableAutoCommit = false
};
var consumeResult = consumer.Consume();
consumer.Commit();
Consumption Positioning
Consumers can set which partition's messages to consume and set the offset.
The example program is as follows:
static void Main()
{
var config = new ConsumerConfig
{
BootstrapServers = "192.168.3.158:19092,192.168.3.158:29092,192.168.3.158:39092",
GroupId = "foo",
// Whether to auto commit, does not affect self-positioning consumption
EnableAutoCommit = true
};
using (var consumer = new ConsumerBuilder<Ignore, string>(config).Build())
{
// Reset this consumer group's offset in a certain partition
consumer.Assign(new TopicPartitionOffset(new TopicPartition("hello-topic", new Partition(0)), new Offset(0)));
consumer.Assign(new TopicPartitionOffset(new TopicPartition("hello-topic", new Partition(1)), new Offset(0)));
consumer.Assign(new TopicPartitionOffset(new TopicPartition("hello-topic", new Partition(2)), new Offset(0)));
consumer.Subscribe("hello-topic");
while (true)
{
var consumeResult = consumer.Consume();
Console.WriteLine(consumeResult.Message.Value);
}
}
}
If you want to start consumption from a specific time, the example is as follows:
static void Main()
{
var config = new ConsumerConfig
{
BootstrapServers = "192.168.3.158:19092,192.168.3.158:29092,192.168.3.158:39092",
GroupId = "foo",
// Whether to auto commit, does not affect self-positioning consumption
EnableAutoCommit = true
};
using (var consumer = new ConsumerBuilder<Ignore, string>(config).Build())
{
var timestamp = new Timestamp(DateTime.Now.AddDays(-1));
// Reset this consumer group's offset in a certain partition
consumer.Assign(consumer.OffsetsForTimes(new List<TopicPartitionTimestamp>
{
new TopicPartitionTimestamp(new TopicPartition("hello-topic", new Partition(0)),timestamp),
new TopicPartitionTimestamp(new TopicPartition("hello-topic", new Partition(1)),timestamp),
new TopicPartitionTimestamp(new TopicPartition("hello-topic", new Partition(2)),timestamp)
}, timeout: TimeSpan.FromSeconds(100)));
consumer.Subscribe("hello-topic");
while (true)
{
var consumeResult = consumer.Consume();
Console.WriteLine(consumeResult.Message.Value);
}
}
}
Conditional Subscription
RabbitMQ has fuzzy subscriptions, but Kafka does not have this feature, so if you want to subscribe to Topics that meet the conditions, you need to first obtain all Topics in the cluster, filter them, and then subscribe to those Topics.
The example code is as follows:
static async Task Main()
{
var adminConfig = new AdminClientConfig
{
BootstrapServers = "192.168.3.158:19092"
};
var config = new ConsumerConfig
{
BootstrapServers = "192.168.3.158:19092",
GroupId = "C",
GroupInstanceId = "C1",
AutoOffsetReset = AutoOffsetReset.Earliest,
};
List<string> topics = new List<string>();
using (var adminClient = new AdminClientBuilder(adminConfig).Build())
{
// Get all topics from the cluster
var metadata = adminClient.GetMetadata(TimeSpan.FromSeconds(10));
var topicsMetadata = metadata.Topics;
var topicNames = metadata.Topics.Select(a => a.Topic).ToList();
topics.AddRange(topicNames.Where(x => x.StartsWith("hello-")));
}
using (var consumer = new ConsumerBuilder<string, string>(config)
.Build())
{
consumer.Subscribe(topics);
while (true)
{
var consumeResult = consumer.Consume();
Console.WriteLine($"key:{consumeResult.Message.Key},value:{consumeResult.Message.Value},partition:{consumeResult.Partition}");
}
}
}
The deserializers, interceptors, and processors in the consumer can refer to the producer in Chapter 4 and will not be elaborated further here.
文章评论